Infrastructure Growth Dominates AI Investment Potential
Key Takeaways
- The evolution of generative artificial intelligence (gAI) has driven significant progress in the pursuit of artificial general intelligence (AGI) and will spark enormous demand for infrastructure investment
- The appetite for building bigger systems with better performance is still supported by multiple “scaling laws”
- The market is grossly underestimating the durability of the infrastructure investment cycle
The early winners in the generative artificial intelligence (gAI) era have largely been infrastructure providers. We expect that trend to persist in the near- and intermediate term as innovators continue to push the boundary of what’s possible. While the first iterations of gAI models have certainly been impressive, there is a long road ahead before we achieve more accurate and practical capabilities, including artificial general intelligence (AGI).
The gAI systems we use today are so-called large language models (LLMs) that are built on neural networks with many layers and up to trillions of parameters. Generative AI is defined by the ability of the machine to create new and unique content. Today’s leading foundation models are seemingly infinitely more capable than models from three years ago but will pale equally in comparison with models that will be common three years from now. An enormous amount of incremental infrastructure investment will be required to keep pushing that innovation boundary. While it will take time for the companies that adopt AI to disrupt their industries, the investment opportunity among infrastructure providers holds more immediate and sizeable potential.
We classify potential investment opportunities into one of three buckets: AI Enablers (the hardware that serves as the infrastructure on which AI systems are built and run), AI Systems (the software that makes up the actual AI models and their associated platforms), and AI Adopters (companies in any sector that are using AI to create a competitive advantage). We believe that most of the opportunities lie within the first two buckets at this time.
Like most new technologies, AI technology will never be “good enough” because the nature of technology is to continuously improve while simultaneously becoming more affordable. Ten years ago, self-driving cars were thought to be on the cusp of reality. Despite rapid improvement in performance, particularly among the AI-powered systems today, we have yet to truly deliver on that promise. AI, of course, has actually been around for decades; it’s the gAI flavor that the world has become infatuated with for the last two years. And gAI is likely to follow a trajectory resembling that of self-driving cars: incremental gains every year will seem meaningful at the time but will quickly feel “old.” Likewise for AI, the never-ending cycle of innovation is driving a never-ending need for more computing power and, with it, more networking, memory, power, cooling, and other data center infrastructure.
Scaling the Opportunity
As a result, spending on artificial intelligence is expected to explode in just the next few years. Technology market intelligence firm International Data Corp (IDC), for example, predicts that worldwide AI spending, which includes AI-enabled applications, infrastructure and related IT and business services, will more than double to $632 billion by 2028. Hyperscale cloud companies already are spending hundreds of billions on AI infrastructure on their own. (See Figure 1.)
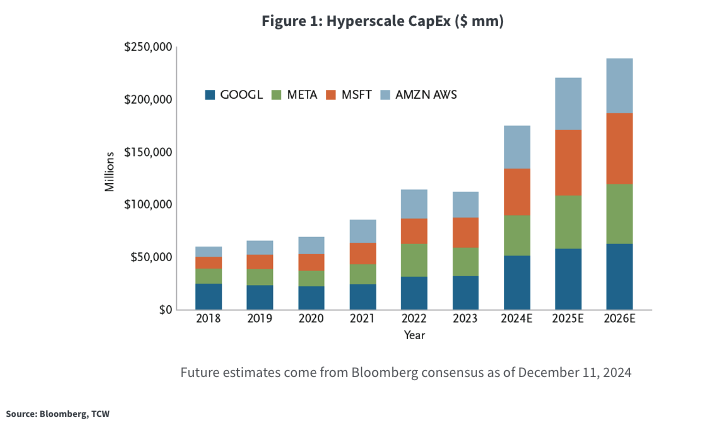
The basis for these projections, and the source of current debate in the industry, is something called “scaling laws.” Scaling laws describe the relationship between underlying resources and the resulting performance gains. In effect, if you keep pulling this lever, things will keep improving, until you can’t pull that lever any longer.
One of the most famous examples of scaling is Moore’s Law, which governed the performance of semiconductor devices for fiveplus decades. Moore’s Law was technically an economic law that projected the number of transistors on a circuit would roughly double every two years for no additional cost – meaning that the cost per transistor would be cut in half every two years. This gave rise to the scaling law of chips; as each transistor got smaller (and cheaper), more performance was packed into each chip for less cost. As a result, a modern CPU powering the computer you are reading this on today is more than 1,000x more powerful than its predecessors from 20 years ago. The scaling laws for AI have multiple pathways for achieving ongoing innovation. Current leading foundation models are amazing, but they can, and soon will be, so much better.
AI scaling is readily apparent in model size. This essentially describes the number of variables in the LLMs. The first version of ChatGPT made available to the public in 2022 was estimated to have 175 billion parameters. The largest models today are already exceeding 2 trillion parameters – a 10-fold increase in just two years. Bigger models have historically performed better, at least in general-purpose applications, and current research suggests we can keep pulling this lever for the foreseeable future. We believe the scaling law of parameter size still holds, which means the world will consume much more compute/infrastructure for the foreseeable future as a means of increasing AI performance.
There are other scaling laws to carry innovation even further. Most of them, such as training data, also require more infrastructure. The first LLMs were trained on a few billion tokens (basically the equivalent of words). By 2022, research showed that training smaller models on more data could produce comparable results to training larger models on less data. Subsequent research showed that training on five times that amount of data was “optimal.” Even more recent studies have shown improved results that now use 2,000 times more data. This scaling law can theoretically continue until a model has consumed all of the data humanity has created.
Another scaling law is around inferencing, which refers to the running of the trained model. Scaling in inferencing has historically been largely a function of the number of users/queries: the more demand, the more supply we would need. This is analogous to cell phones – the more users we have, the more towers we need. Recently, some gAI models have started to incorporate “thinking tokens” into their inference. Basically, the model starts to formulate an answer and then, part way through, pauses and “thinks” about what it’s come up with so far to see if it makes sense. If yes, continue; if no, start over. This gives gAI the first hints of logic and reason. The cost of all these thinking tokens is greater capacity required per query – and that means more infrastructure. It so far appears that the more time (and resources) you spend on thinking inference, the better the performance.
A third driver of infrastructure has more to do with scale than scaling. We envision a world where eventually billions of people interact with AI dozens, if not hundreds, of times per day. The amount of capacity required to achieve that kind of integration in our daily lives will require orders of magnitude more infrastructure investment. This is why some companies talk about a 20-year investment cycle for AI infrastructure valued in the trillions of dollars.
All Roads Lead to Infrastructure Demand
Ultimately the question of whether infrastructure investments have plateaued or not comes down to whether you believe gAI is already as good as it can ever be, and whether you believe “everyone” will use it. We obviously support the latter. And it’s worth repeating that every new GPU requires an incremental investment in memory, networking, power, cooling, security, etc. There are certainly challenges to realizing the dream. Most acutely, there is a shortage of GPU capacity to meet current demand. Power and cooling requirements are also a challenge.
If the scaling laws around model size have already reached their termini, it could be tempting to think that we won’t need increasing amounts of infrastructure, or at least that the bolus is behind us. But the other methods that can improve performance of AI models will still require increased infrastructure investment. In fact, all roads toward AGI currently lead to increasing investment in infrastructure. And, once the models are truly “good enough,” building out the capacity to meet the demand of ubiquitous integration will drive even more infrastructure investments.
We think the market is grossly underestimating the durability of the infrastructure investment cycle. Eventually, and it may be sooner rather than later, the pool of investment opportunities among AI Adopters will also present compelling investment potential. Until that happens, however, we believe that the hardware and software systems that are critical to AI growth – what we call the AI Enablers – are the most attractive areas for investment in the AI universe. The benefit of an active management strategy is that we can shift the portfolio toward AI Adopters when appropriate.
This material is for general information purposes only and does not constitute an offer to sell, or a solicitation of an offer to buy, any security. TCW, its officers, directors, employees or clients may have positions in securities or investments mentioned in this publication, which positions may change at any time, without notice. While the information and statistical data contained herein are based on sources believed to be reliable, we do not represent that it is accurate and should not be relied on as such or be the basis for an investment decision. The information contained herein may include preliminary information and/or "forward-looking statements." Due to numerous factors, actual events may differ substantially from those presented. TCW assumes no duty to update any forward-looking statements or opinions in this document. Any opinions expressed herein are current only as of the time made and are subject to change without notice. Past performance is no guarantee of future results. © 2024 TCW